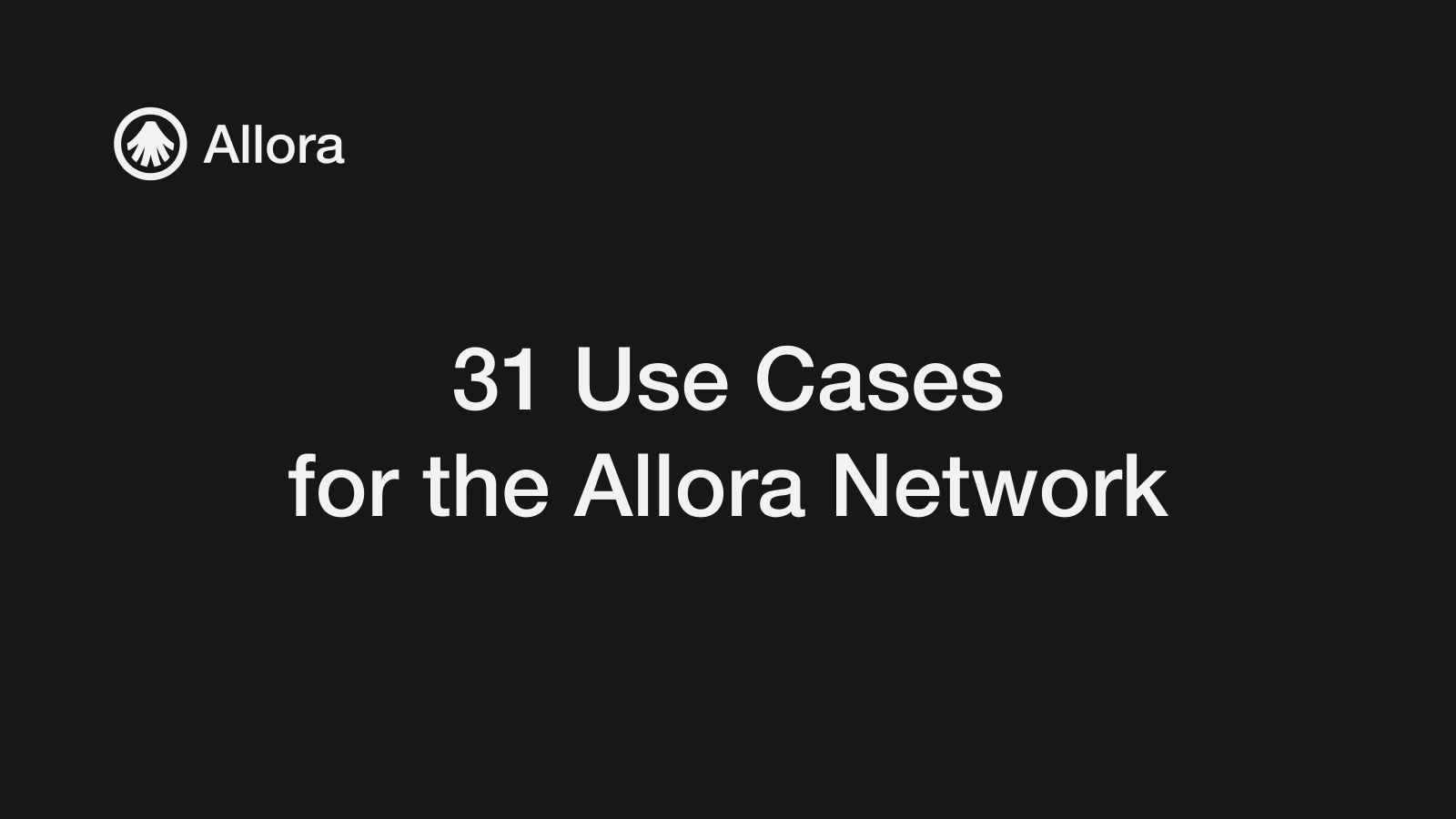
Decentralized AI has the potential to turn opaque, centralized black-box AI systems into open networks that coordinate machine intelligence. However, historically, a few industry monoliths have monopolized AI development, blocking its integration into decentralized environments and skewing public perception. Recent advances like zkML and federated learning have certainly paved the way for embedding secure, trustless AI into decentralized applications.
Yet decentralized AI still faces the barrier of ensuring both continuous self-improvement of models over time, as well as establishing irrefutable trust in any given AI-generated output. This two-sided problem — ensuring trustless AI while maintaining maximum adaptability — has been a sticking point preventing seamless, scalable integration of AI into decentralized networks.
As a self-improving decentralized AI network, Allora establishes a reliable, cryptographically verifiable foundation for AI computations, solving the two-sided problem that has hampered AI until now. Moreover, Allora implements an incentive structure that rewards models on the network for the accuracy of their outputs relative to their specialized topics. As a context-aware network, Allora is able to evaluate model outputs against two factors: real-world conditions and peer models. In other words, the network determines the optimal model outputs for given specific situations.
Rather than acting as isolated components, models built on Allora continuously learn from peer performances over time. This emergent meta-intelligence, which is greater than any individual predictor, recursively adapts to new data meaning the combined efforts of multiple models yields more accurate inferences than any one could.

The foundational model built on the Allora Network, the Upshot pricing model, has distinguished itself for almost 3 years with its precision in asset valuation, consistently delivering valuations for over 400 million assets with an accuracy between 95–99%. The Allora Network is much more than a single model; it is a means of building self-improving, decentralized, objective-focused AI networks, each operating independently to ensure system robustness and scalability, while continuously learning from each other at the same time.
Allora’s initial solutions:
- AI Price Feeds: Allora’s AI price feeds provide dynamic, real-time pricing for a wide array of assets, underpinning the market with reliable, data-driven insights that enhance transparency and liquidity.
- AI-Powered Vaults: Allora’s AI-powered vaults offer bespoke, intelligent asset management solutions that adapt to market conditions and optimize returns, with no human management necessary.
- AI Risk Modeling: Allora’s sophisticated AI risk modeling tools offer nuanced, predictive insights, enabling more informed decision-making and robust risk mitigation strategies.
- AnyML: AnyML democratizes access to advanced machine learning, allowing users to integrate any AI or ML model into their products.
At its core, Allora provides developers with an essential toolkit: everything you need to create decentralized AI applications. This includes federated learning frameworks, zkML integration tools like the zkPredictor, adaptive model aggregation methods, decentralized incentive structures, security assurances, and analytics dashboards. With this versatile stack, developers can ideate, refine, and deploy a multitude of use cases, some of which are outlined below.
To learn more, or if you’re eager to dive deeper, join our Discord, follow us on Twitter, and explore our docs to start integrating decentralized AI today.
DeFi
DeFi has transformed how we access and leverage financial services. Exchanging value and assets through decentralized protocols — rather than traditional institutions — enables the permissionless creation of complex financial products. Until crypto came along, a few influential entities controlled the monetary systems. This concentration of centralized control had, for decades, prohibited universal access to advanced investment tools.
The Allora Network utilizes technologies like zkML and federated learning to chart a new course for DeFi. Decentralized AI plays a pivotal role in crafting advanced investment tools, especially those involving real-time data analysis, AI-powered insights for dynamic valuations, risk assessment, and predictive analytics. With these capabilities, financial products built on Allora are more efficient, transparent, and accessible than centralized frameworks.

1. AI-Powered Reward Strategies
Current DeFi return strategies often struggle with real-time market responsiveness and are limited in their ability to process vast datasets, resulting in less optimized returns. AI, on the other hand, can analyze data with speed and efficiency that no human could replicate, overcoming these limitations.
Based on these analyses, DeFi protocols like RoboNet could implement an AI model that develops dynamic return strategies, involving a combination of buying, holding, and selling at specific intervals or price points. This strategy would then be executed automatically to optimize for maximum return, ensuring anyone has access to institutional-grade investing strategies.
2. AI-Enhanced Prediction Markets
Traditional prediction markets often suffer from low liquidity, especially in niche areas. AI agents can overcome these limitations by efficiently managing market making and trading strategies. AI agents, being more efficient than human traders, can significantly increase trading volumes. This higher activity boosts liquidity, making markets more efficient, all while delivering more accurate projections across niche events.
Prediction markets could build on Allora Network to create AI agents that specialize in various fields, analyzing vast datasets including historical trends, current events, and nuanced market indicators, and then trade in different prediction markets based on those analyses. This leads to more granular predictions that were previously unattainable. These agents could then manage on-chain DeFi vaults, using deposited funds to trade across relevant prediction markets autonomously, thus facilitating dynamic market making and trade execution to bolster liquidity and volume.
For a more in-depth analysis of how AI agents would work in prediction markets, read Upshot CEO Nick Emmons’s post here.
3. AI-Optimized Liquidity Management on Perp DEXs
Perp DEXs rely on active and granular liquidity management to function effectively, which has historically led to inefficiencies, such as slippage, delayed responses to rapid market movements, and difficulty in maintaining optimal order book depth. However, Allora’s AI price feeds introduce necessary liquidity optimization capabilities to perp DEXs.
AI can analyze market conditions in real-time, predicting periods of high volatility or trading volume. Based on these predictions, the AI can dynamically adjust the liquidity in the order book. This means maintaining a healthy ratio of buy and sell orders, which is crucial for market stability, thereby safeguarding value for traders by minimizing slippage from sudden market movements.
Market makers on platforms could further elevate their offerings by integrating Allora’s AI-powered price feeds for liquidity optimization. By predicting periods of peak volatility, liquidity providers can preemptively adjust liquidity parameters across their decentralized order books, upholding balanced order ratios aligned with upcoming conditions — essential for platform equilibrium and minimal slippage.
4. AI-Powered Price Oracles for Long-Tail and Real World Assets
Long-tail assets such as physical and digital collectibles have traditionally faced pricing challenges due to their unique nature and low market liquidity. However, Allora’s AI price feeds enable accurate, reliable valuations tailored to each asset’s unique traits. These advanced oracles overcome the limitations of traditional price oracles by extracting insights from a wide range of sources beyond just market prices, including social media sentiment, news articles, and historical trends, ensuring a comprehensive and reliable valuation mechanism.
Platforms like Watches.io and NFTfi are already pioneering the use of AI in long-tail price oracles. Watches.io leverages Allora’s AI to underpin its watch-backed NFT financialization, providing a secure and transparent pricing mechanism that supports a variety of financial derivatives, such as NFTfi’s tokenized watch lending. This same process could apply to other RWAs such as real estate, sneakers, and rare collectibles.
5. Automated On-Chain Index Funds
Automated On-chain Index Funds offer a modern twist on the traditional index fund by eliminating the need for costly fund managers. Instead, through AI-driven asset reallocation, AI could continuously monitor the market and rotate investments among various assets, such as the top 20 tokens across crypto, niche sectors like small cap tokens, or NFT indices. The use of AI not only optimizes returns based on comprehensive market analyses, but also significantly reduces the barriers to sophisticated investment strategies.
Automating index fund allocation could allow platforms to offer investors the advantage of a dynamically-managed portfolio, which adapts instantaneously to market fluctuations. This innovation makes dynamic, sophisticated investment strategies more accessible, removing the necessity for manual oversight, and therefore cutting out traditional index fund management fees.
6. AI-powered Maximal Extractable Value
AI will transform how transactions are optimized on-chain. AI could be used to predict future actions of crypto investors, which would enable the execution of advanced MEV strategies that go beyond current simulation-based methods, including transaction reordering, insertion, and the creation of new transactions. By harnessing probabilistic and multi-block profit-extracting strategies, AI-powered MEV bots could identify and exploit profitable opportunities with unprecedented precision and efficiency.
For block builders and searchers, AI agents could directly execute or recommend specific actions to capitalize on arbitrage opportunities by reordering or creating transactions. This precise, real-time strategy enhancement could significantly boost the efficiency and profitability of trades on these DEXs.
7. AI-powered Swap Aggregation and Transaction Routing
AI-powered swap aggregation and transaction routing addresses the limitations of current DEX methodologies by introducing real-time adaptability to optimize trading for factors such as fees, slippage, and liquidity. Leveraging AI to analyze vast amounts of data across multiple platforms would help identify the most efficient transaction paths, surpassing traditional static routing methods. An AI agent, capable of evaluating liquidity, transaction fees, slippage rates, and available trading pairs in real time, ensures that trades are executed in the most advantageous manner possible.
For a DEX aggregator, AI-enhanced routing could continuously scan and assess possible swap routes across a myriad of DEXs. The AI could then pinpoint the optimal path that minimizes costs and maximizes returns for the trader. The result would be an automated, smart contract-executed swap, following the AI-recommended route, thereby streamlining the trading process and ensuring traders receive the best possible deal with every transaction.
Here’s how this might work in practice:
- A trader wants to swap one token for another on a DEX.
- The AI agent evaluates all possible swap routes across multiple DEXs.
- It identifies an optimal route that minimizes fees and maximizes return.
- The swap is executed automatically via smart contracts, following the AI-recommended route.
8. Intent-Based Blockchain Solvers Using AI
Unlike traditional solvers that operate on explicit commands, AI-powered solvers can understand and efficiently execute complex user intents, even when dealing with nuanced requests. Instead of merely executing transactions based on explicit commands, “intents” allow users to delegate transaction construction and execution to new actors called “solvers.” AI models equipped with natural language processing and context-awareness can interpret these intents with a level of nuance far beyond basic instructions.
DeFi platforms could all users to employ AI-driven solvers to uncover intelligent ways to fulfill different intents. For example, an intent such as “I want to buy 10 ETH for no more than $3,500 per ETH” could be addressed by an AI solver that assesses a wide range of market opportunities, potentially taking on minimal risk to maximize profit and value. This would allow DeFi protocols to offer more personalized and efficient financial services, ensuring transactions are executed in alignment with users’ trading goals.
9. Advanced Loan Underwriting
Loan underwriting is largely governed by straightforward logic encoded in smart contracts, which often lacks the depth and flexibility needed for complex risk assessments. AI can develop more advanced, capital-efficient lending systems, offering nuanced evaluation of risk factors. This approach leverages AI to evaluate borrower credibility, assess collateral values for RWAs, and adapt to changing market conditions. This would elevate capital efficiency and align lending terms more closely with the nuanced needs of borrowers.
Lenders could significantly enhance their lending framework by incorporating Allora’s AI risk modeling solution. This integration would allow for a more granular assessment of collateral and borrower reliability, facilitating the customization of loan conditions such as interest rates, loan-to-value ratios, and repayment schedules. Setting terms that accurately reflect the risk and borrower’s circumstances could expand credit access.
10. Decentralized Bond Issuance and Valuation
Current bond issuance and valuation face inefficiencies, limited accessibility, and insufficient real-time data analysis. These issues have long plagued the bond market. AI could meticulously assess bonds based on market conditions, issuer creditworthiness, interest rates, and projected returns.
Municipal governments could fund an infrastructure project through bond issuance using an AI agent that evaluates the government’s financial health, the project’s viability, and prevailing market conditions to accurately determine the bond’s value and subsequently set a competitive interest rate. Investors could then purchase these bonds directly on a DeFi platform, with all terms efficiently managed via smart contracts. Moreover, the AI agent could continuously reevaluate the bond, making the bond issuance process streamlined, transparent, and dynamic.
11. AI-Powered Insurance Vaults
The insurance industry spends too many resources settling claims. Their processes are bogged down by manual administration and slow decision-making. AI could revolutionize this sector by automating these processes and ensuring more accurate and quicker settlements. By employing AI for the automation of policy management in decentralized vaults, the process from claim submission to final settlement becomes not only faster but also more precise.
AI could automate every aspect of the insurance lifecycle — administration, tracking, and updating of policies, alongside assessing and settling claims based on rigorous, predefined criteria. Insurance companies already use predictive models to estimate hurricane damage, for example. But imagine an AI-powered insurance product that, based on sophisticated risk models, could pay out a percentage of a hurricane claim on day 0, even before the storm clears. The result would be a much more efficient process, for both the insurance company and the insured.
DAOs
DAOs theoretically allow for a more agile and responsive governance model, where decisions reflect the collective will rather than the interests of a few. Yet, in practice, DAOs face significant challenges, including inefficiencies in processing vast amounts of information for decision-making, and difficulties in achieving true consensus. This can lead to governance paralysis and reduced operational effectiveness. The lack of advanced analytical tools to sift through proposals and predict their outcomes has often left DAOs struggling to fulfill their governance potential.
Integrating decentralized AI can automate the evaluation of proposals based on set criteria, predict the outcomes of these proposals based on historical data, and provide a more nuanced analysis of the potential impacts of these proposals to enhance the DAO’s decision-making processes.

12. DAO Treasury Management
DAOs struggle to accurately predict the outcomes of their treasury decisions. By leveraging AI, DAOs can analyze data to predict cash flow, investment returns, and financial risks, facilitating more strategic fund allocation and management decisions.
DAOs could utilize AI-powered analytical models to enhance its treasury management. These models would enable the platform to make data-driven decisions regarding investments, budgeting, and liquidity management. This approach not only aligns with the transparency and trust that DAOs strive for, but also ensures the treasury’s resources are managed more efficiently, safeguarding the organization’s financial health.
13. AI Agents as Governance Participants
AI agents could solve the widespread issue of voter apathy in DAOs. These agents would introduce a means for precise, data-driven participation, thereby combating voter apathy and the frequent misalignment between DAO delegates and their constituent interests. The absence of such technology has previously left DAOs and political systems alike struggling to maintain active and representative governance structures.
AI agents could participate in governance decisions, automatically voting on proposals or aiding in the formulation of policies. These AI representatives would be trained on the individual political preferences and values of their constituents, enabling them to automatically vote in alignment with these interests. These agents could potentially represent the specific preferences of their constituents more accurately than human representatives. This automated involvement would ensure all decisions are consistently aligned with the community’s interests. Ultimately, this would foster a governance environment that is more engaged and in tune with the collective will.
Gaming
Integrating AI in gaming could deliver experiences that are not only more immersive but also deeply personalized, shaping games that learn from and adapt to each player’s unique skills and desires. Yet, the reliance on centralized AI systems has introduced significant challenges, including concerns over privacy, and the inability to dynamically evolve game worlds in real-time with player inputs. Consequently, players are often left with experiences that feel static and disconnected from their actions.
Decentralized AI offers a means to create more adaptive game mechanics and even real-time, in-game economy adjustments. This approach not only enhances security for players but also unlocks new possibilities for game personalization, making every player’s experience unique. Through the application of decentralized AI, games can become dynamic environments that respond to the diverse preferences of their global player base.

14. Personalized Gaming Experiences with AI
Player individuality is often neglected in gaming experiences, leading to static and one-dimensional gameplay. AI offers a solution by enabling games to adapt to individual play styles and choices. The main obstacle to achieving this level of personalization has been the absence of technologies capable of understanding and adapting to the nuanced preferences of each player in real-time.
Game studios could integrate AI agents that analyze player interactions, decisions, and performance to dynamically adjust game elements such as difficulty levels, story paths, and in-game events. This ensures a gaming experience that is uniquely tailored to each player. Moreover, AI could continuously learn from each player’s actions so the game can transform in alignment with the player’s evolving style, ensuring a consistently fresh and engaging experience that promotes long-term player retention.
15. AI-Optimized Blockchain Gaming Strategies
Historically, one-size-fits-all gaming strategy recommendations fail to account for individual player behaviors and the dynamic nature of game worlds. In this context, AI is capable of delivering personalized, real-time strategic insights that evolve alongside the game and its players. Traditional strategy guides have struggled to adapt to the unique playing styles of each player, leaving a gap that AI is uniquely positioned to fill.
Based on in-depth analysis, AI could provide recommendations to players on how to optimize their gameplay. And for games involving trading or other in-game assets, AI could even analyze market trends and asset values, providing players with insights for optimal asset management.
The result is a gaming ecosystem where each player’s journey is distinct, supported by AI-driven insights that maximize engagement and strategic success.
16. AI-Enhanced Virtual Economy Management
Gaming economies struggle to battle inflation or resource depletion impacting the player experience. These issues make economic stability within games a paramount concern. AI introduces a level of adaptive control and predictive capability that enables the potential to maintain a balanced and engaging virtual economy that evolves in response to player actions and market dynamics.
In a blockchain-based MMORPG where players trade NFTs and in-game tokens, an AI model could play a crucial role in monitoring the economic pulse of the game world. By continuously analyzing transaction data, the AI could identify trends that may indicate the onset of inflation or other economic imbalances. Upon detecting these trends, the AI could implement countermeasures, such as adjusting the drop rates of valuable items or modifying transaction fees, to preserve economic equilibrium. This AI-driven approach ensures a virtual economy that dynamically adjusts to remain stable and fair for all players.
17. Enhanced NPC Interactions
Non-player characters are a critical component for immersive storytelling and player engagement in video games. By improving the intelligence and responsiveness of NPCs, players can experience more realistic and meaningful interactions — where NPCs can learn from, remember, and react to player actions. Traditionally, the complexity of creating such dynamic NPC behavior has been limited by the capabilities of static programming models, unable to truly adapt to or reflect the nuanced decisions of players.
Integrating AI enables new possibilities for developers looking to breathe life into their virtual worlds. Imagine NPCs with advanced NLP and machine learning capabilities, enabling them to understand and respond to player choices in a more nuanced and human-like manner. Consequently, NPCs would become dynamic participants in the game narrative, capable of forming relationships with players and altering the course of the game based on these interactions. This would enhance the depth of the gaming experience and ensure that each player’s journey is unique, fostering a deeper connection to the game world and its inhabitants.
18. Enhanced Movement Validation for Move-to-Earn Economies
Movement validation in move-to-earn platforms is necessary to maintain a trustworthy and healthy economy. AI and zkML technology could be used to ensure that rewards are fairly distributed based on genuine activity, encouraging more consistent and honest participation. Previously, the challenge has been accurately verifying the authenticity of physical movement without invasive tracking or easily spoofed systems.
However, move-to-earn platforms can use zkML technology like Allora’s zkPredictor to enable a secure, privacy-preserving validation process. zkML, or zero-knowledge machine learning, allows the system to verify physical activities without exposing any individual user data. This ensures that rewards are distributed fairly, based on verified activities, and combats fraudulent claims of physical activity.
19. AI-Powered Anti-Cheat
As games have become more financialized, cheating has become more lucrative than ever. Players even use bots to grind for items and sell them online. Entire businesses have emerged to farm items and sell them to players, while other cheats like aimbots and teleportation hacks can completely ruin the gaming experience. There are anti-cheat systems in use today- — they work by running a program on the player’s computer to check for suspicious activity. But it’s an endless game of cat and mouse, with hackers finding ever-effective means of circumventing detection, such as running cheats on a separate laptop connected to their gaming PC.
With players in web3 games owning their in-game items as NFTs, the incentive to cheat will only grow. AI could be an effective means of catching and preventing these cheats. By analyzing player behavior and transactions, AI systems could identify irregularities that suggest cheating. For example, AI could flag players who fall outside the expected bell curve for matches won, time played, in-game currency earned, and other behaviors. It could also detect bots that grind low-level content for long periods of time without ever going offline. With AI monitoring for these red flags, game publishers could take swift action against cheaters, ensuring a fairer and more enjoyable gaming experience for all players.
Renewable Energy
Using renewable energy sources symbolizes a critical step towards sustainability, aiming to reduce carbon emissions and a global reliance on fossil fuels. However, integrating renewable sources like solar and wind presents challenges, including variability in power generation and difficulties in predicting supply and demand. Traditional systems have struggled with these issues, often leading to inefficiencies in energy distribution and storage, and ultimately, underutilization of renewable resources.
Decentralized AI could enable more accurate forecasting and real-time management of renewable energy systems. For instance, AI could predict the energy production from solar and wind sources based on weather data, adjusting grid operations to match supply with demand. Additionally, AI price feeds could optimize energy trading and storage decisions, ensuring energy is distributed at the most cost-effective times. This approach enhances the efficiency of renewable energy systems and supports a transition to a more resilient energy infrastructure.
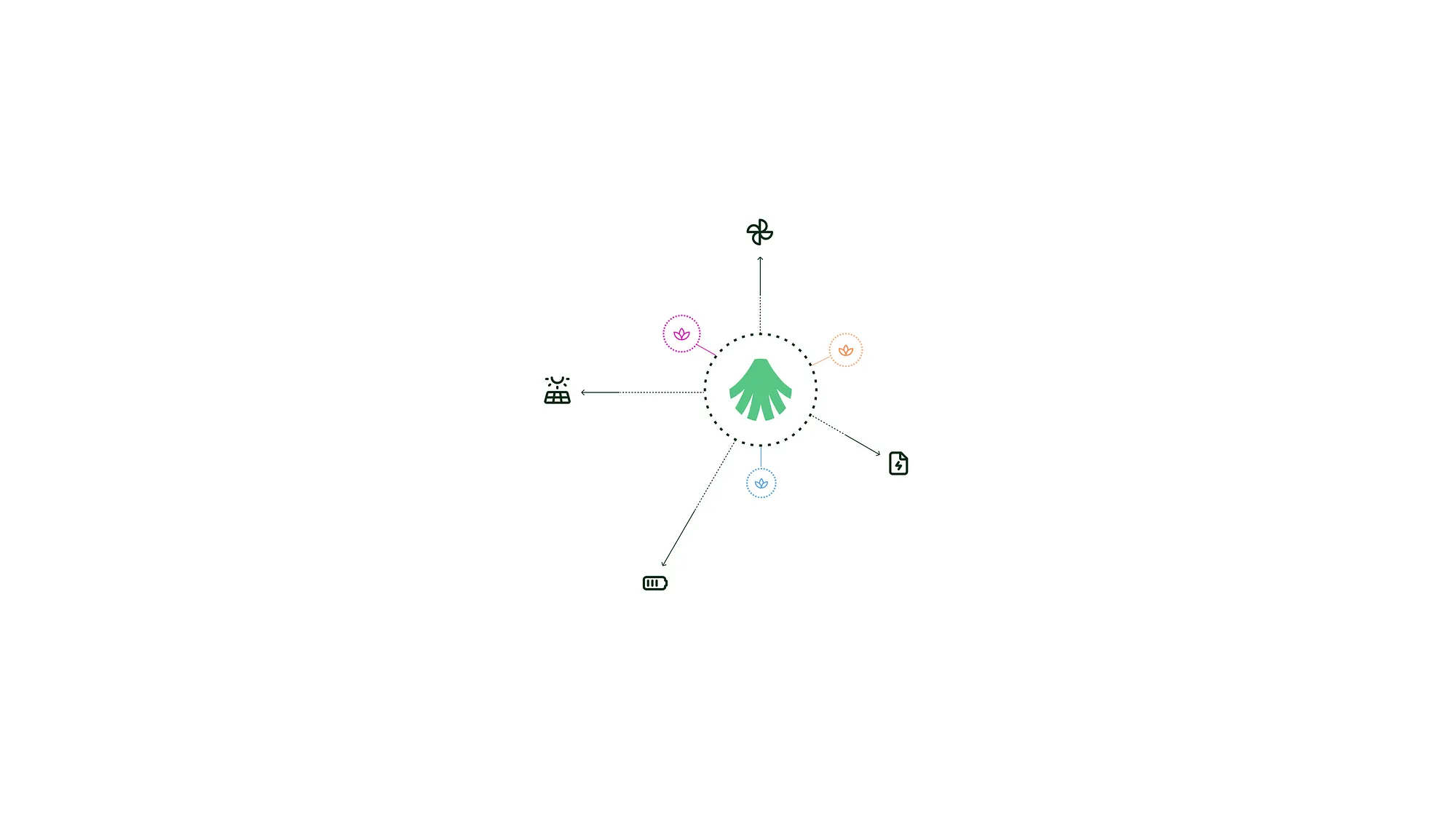
20. Decentralized Carbon Credit Marketplace
A decentralized carbon credit marketplace would allow businesses and individuals to trade carbon credits in an effort to offset their carbon footprint, promoting environmental sustainability. However, the current carbon credit market faces challenges in verifying the authenticity of credits and inconsistencies across market standards. These issues have historically hindered the effectiveness and trust in carbon offset initiatives.
Integrating AI for data validation and analysis, a decentralized carbon credit marketplace could overcome these obstacles. AI agents could rigorously validate carbon credits by analyzing project data against environmental standards and tracking the lifecycle of each credit from issuance to retirement. Moreover, leveraging AI for market analysis and regulatory oversight could offer investors predictive insights, enhancing their decision-making process regarding carbon credit investments. This would ensure that every credit traded represents a genuine contribution to carbon reduction efforts.
21. AI-Optimized Renewable Energy Distribution
Unlike traditional energy systems that often struggle to integrate and optimize multiple renewable sources, AI could be used to dynamically manage the distribution of energy across a decentralized network. The challenge with integrating diverse renewable sources into the energy grid has been ensuring that energy is not only produced sustainably but also distributed in a manner that aligns with fluctuating demand and supply conditions.
Imagine a smart city powered by a decentralized grid of renewable energy sources, including solar panels and wind turbines. In this city, an advanced AI system is tasked with the continuous monitoring and management of energy production and distribution. This system analyzes data in real-time to adjust energy flows, directing excess production into storage units during times of low demand, and efficiently utilizing stored energy when production is low. This AI-driven approach to energy distribution would ensure maximum efficiency, significantly reducing waste and improving the reliability and sustainability of a city’s energy supply. The result is a more resilient energy infrastructure capable of meeting the city’s needs while maintaining sustainability.
22. Energy Consumption Optimization for Crypto Mining
The intensive energy consumption of crypto mining has raised environmental concerns for years. Employing AI models to scrutinize and enhance various facets of the mining process represents a promising solution to these challenges. Traditional mining operations often overlook the potential for optimizing hardware performance, cooling systems, and algorithm efficiency, leading to unnecessary energy expenditure and elevated costs.
A crypto mining farm could deploy AI to continuously monitor the performance and energy consumption of its mining rigs. This AI system would evaluate the energy efficiency of each rig, pinpointing those with suboptimal energy usage. Following this analysis, adjustments could be made to improve energy efficiency, such as optimizing mining parameters or scheduling maintenance. Additionally, the AI could strategically time mining operations to coincide with periods of lower energy prices or higher availability of renewable energy, thereby reducing operational costs. This strategic application of AI makes crypto mining more environmentally friendly, and also ensures it remains economically feasible.
23. Optimizing Energy Use in Buildings
AI could be used to dynamically adjust a building’s energy consumption based on real-time data such as occupancy, weather conditions, and more, significantly reducing energy waste. The challenge has historically been the lack of responsive and intelligent building energy systems capable of processing and acting on diverse data streams in real time, resulting in excessive energy use even during low occupancy periods.
A building equipped with AI-driven smart technology could monitor its energy use and automatically adjust heating, cooling, and lighting based on current needs. For instance, during periods of low occupancy, the system would reduce energy expenditure, whereas, on colder days, it would optimize heating to maintain comfort without waste. Through the implementation of such AI solutions, buildings can become more sustainable and cost-effective.
Social/Consumer
Our reliance on centralized systems for our social and consumer actions online has led to significant issues, including privacy concerns, data misuse, and a one-size-fits-all approach that often misses the mark on true individualization. The result is a landscape where users are increasingly wary of how their data is used and skeptical of the personalization they receive, eroding their trust in the very platforms designed to serve them.
Decentralized AI could yield a new era of privacy-respecting, personalized social and consumer experiences, all while ensuring individuals retain control over their data. This shift opens up new possibilities for genuinely customized experiences, setting a new standard for how social and consumer applications operate.

24. Decentralized Credit Scoring
Traditional credit scoring systems often fail to capture the full picture of an individual’s financial health, relying on opaque criteria that can introduce biases. Decentralized AI offers a pathway to more accurately assess creditworthiness by analyzing a wide array of financial behaviors directly onchain, resulting in a more nuanced and fair evaluation of financial health.
For instance, when a user applies for a loan on a DeFi platform, the platform’s AI can analyze their transaction history, including payment patterns and asset diversity, to assign a dynamic credit score. This score, grounded in transparent and verifiable data analysis, would then be used to determine the user’s loan eligibility and terms, ensuring fair and unbiased results. The continuous update of this score, based on evolving financial behaviors, would also ensure an up-to-date reflection of the user’s credit status. Decentralized credit scoring could democratize access to financial services, ensuring that individuals are assessed based on their actual financial practices rather than a narrow set of traditional criteria.
25. Real-time Sentiment Analysis for Trading
Traditional market analytics struggle to capture shifting market sentiments in real-time. Using AI to determine market sentiment would allow traders to leverage a multitude of data inputs, providing a nuanced view of market attitudes toward specific assets or the broader financial landscape. Previously, the sheer volume and variety of data required for effective sentiment analysis made real-time insights difficult.
For instance, traders could utilize AI to monitor and analyze sentiment on social media and financial news platforms, capturing the collective mood regarding cryptocurrencies or stocks. This AI-driven insight would allow traders to adapt their strategies immediately, perhaps even triggering autonomous AI agents to trade on their behalf based on this sentiment analysis.
26. Automated Content Moderation for Decentralized Social Networks
As decentralized networks grow, so does the challenge of distinguishing between human-created content and AI-generated content. Traditional methods fall short in accurately identifying AI-generated content due to its increasingly sophisticated nature. Leveraging advanced AI could help discern subtle differences that distinguish genuine content from AI-generated material.
Implementing AI detection enables real-time identification and management of AI-generated content, ensuring users are informed about the origin of the content they consume. This use of AI not only helps in maintaining the authenticity of content but also supports transparency regarding the use of AI in content creation.
27. Synthetic Identity Detection
Synthetic identity fraud involves creating identities using a mix of real and fake information, an increasingly difficult form of fraud to detect. The primary hurdle has been the lack of advanced, scalable tools capable of analyzing the depth of data needed to identify these fabricated identities accurately.
A financial institution could utilize AI to cross-reference new account applications against a comprehensive database of known patterns associated with synthetic identities. Analyzing discrepancies in application details, transaction behaviors, and credit usage patterns that deviate from the norm would allow the institution to flag potential synthetic identities for further review. This reduces the risk of financial loss due to fraud and streamlines the account verification process.
28. AI-Powered Crypto Portfolio Tracker
Current crypto portfolio trackers only provide a superficial view of holdings without in-depth risk analysis or strategic advice. An AI model could assess a specific portfolio’s risk based on its performance against market benchmarks, and even provide strategic insight for portfolio optimization or even copy-trading.
For example, by analyzing a portfolio’s historical performance data, current market trends, and individual asset volatility, AI can suggest strategies for rebalancing, diversification, or exploring new investment opportunities tailored to the user’s risk tolerance and goals. Beyond mere tracking, this AI model could allow users to emulate the strategies of successful portfolios, thereby democratizing access to expert investment tactics.
29. AI-Driven Financial Behavioral Analysis
Traditional financial analysis often struggles to capture the full scope of an individual’s financial behavior, missing out on opportunities for personalized service. AI could analyze each customer’s financial transactions, behaviors, and preferences, such as how they spend, invest, save, and their approach to risk. This detailed analysis has been largely impractical in the past due to the complexity of data and the need for real-time adaptability.
Implementing AI models allows financial institutions and DeFi platforms to tailor their services precisely. For instance, after evaluating a user’s spending habits and investment activities, a platform could recommend customized investment products, suggest budgeting strategies, or offer loan options with personalized interest rates. This level of customization could potentially increase user engagement and loyalty to the platform.
30. Predictive Healthcare
AI’s role in healthcare, particularly in disease prediction and diagnosis, marks a pivotal shift towards early and precise medical interventions. The crux of this advancement lies in AI models’ capacity to digest and interpret extensive datasets — ranging from electronic medical records and genetic sequences to detailed imaging studies. This depth of analysis facilitates the identification of disease markers well before conventional methods might catch them, offering a significant edge in the early detection of conditions like cancer.
For example, AI could be used to analyze x-ray images for the early detection of cancer. Traditional review processes might miss early or subtle signs of cancer, especially in dense tissue. An AI model, trained on billions of x-rays, can detect minute irregularities indicating early-stage cancer, often before they become clinically apparent. This leads to earlier intervention, potentially improving patient outcomes by starting treatment sooner. Through such applications, AI optimizes the diagnostic process.
31. AI-Powered Supply Chain Optimization
Optimizing supply chain shipping routes, package placement, and shipping frequency is a complex task. Current supply chain management systems, although advanced, often struggle to keep up with ever-increasing demands.
AI has the potential to improve supply chain optimization by analyzing vast amounts of data such as fuel costs, delivery deadlines, parcel dimensions, and more to determine the most efficient way to pack and transport goods. Furthermore, AI can be applied to assembly lines, predicting which parts need to be replaced and subsequently automatically ordering and shipping them to prevent downtime.
About the Allora Network
Allora is a self-improving decentralized AI network.
Allora enables applications to leverage smarter, more secure AI through a self-improving network of ML models. By combining innovations in crowdsourced intelligence, federated learning, and zkML, Allora unlocks a vast new design space of applications at the intersection of crypto and AI.
To learn more about Allora, visit the website, Twitter, Discord, and developer docs.
To apply to the Allora Network Community Advocate Program, fill out this form: