
Allora is built on the desire to create a world where machine intelligence improves humanity by offering unique and actionable insights that outperform all other forms of inference. In this world, machine intelligence is openly accessible and transparent, inviting contributions from anyone with data or algorithms that improve the network.
While other AI networks may share these intentions, Allora achieves this goal via two defining mechanisms:
✳️ Context-Awareness
Allora is built with the understanding that selecting the best inference across an AI network often depends on contextual details that themselves may require machine intelligence to be identified.
✳️ Differentiated Incentives
Allora's architecture acknowledges that different roles within a network––in our case, workers, reputers, and validators––require different incentive structures to ensure optimal performance and fair reward distribution.
Let’s break these two factors down in detail.
What Makes Allora Context-Aware?
Allora’s context awareness is facilitated by the network rewarding participants’ forecasts of each other’s performance under certain conditions. In many networks, worker nodes only provide an inference and nothing else.
On Allora, worker nodes have the capability of providing two outputs:
- An inference
- A forecast of the accuracy of each others’ inferences
In other words, the network supports a logic whereby worker nodes are rewarded for commenting on each other’s expected performance. This works by something called forecast-implied meta-inference. This is the core mechanism that makes Allora context-aware.
For example, for a specific ML objective on Allora, called a topic (analogous to a sub-network), that aims to predict the price of BTC, other workers might comment their awareness that an individual model performs worse when US equities markets are closed.

How Allora’s Inference Synthesis Process Works
Like their counterparts in other decentralized AI networks, workers in Allora provide their inferences to the network. What sets Allora apart is the additional responsibility of the forecasting task: workers forecast the accuracy of other participants’ inferences within their specific topic. This dual-layer contribution significantly enriches the network’s intelligence.
The entire process of condensing inferences and forecasted losses into a single inference is referred to as Inference Synthesis. Here’s how the Inference Synthesis mechanism unfolds:
- Workers Provide Loss Forecasts—Workers estimate the potential losses (or inaccuracies) of models submitted by their peers in the same topic.
- The Network Weights According to These Forecasts—The network assesses these forecasted losses, applying weights to workers’ inferences based on their expected accuracy. A lower forecasted loss means higher accuracy, warranting a higher weight, and a higher forecasted loss means lower accuracy, warranting a lower weight.
- The Topic Optimizes Model Contributions—The topic doesn’t simply favor the models with the lowest forecasted losses. Instead, each topic intelligently combines elements from various contributions—taking, for example, 80% from one worker’s model and 20% from another’s—to craft a single, robust forecast-implied meta-inference.
- The Network Combines All Inferences—The forecast-implied inferences are then combined with all other inferences, taking into account their historical performance, to formulate a comprehensive, topic-wide meta-inference. This method ensures the network leverages the most effective inferences, thereby always outperforming the individual models in the network.

How Allora’s Differentiated Incentive Structure Works
In the Allora Network, one cannot buy the truth. However, one can be—and should be—rewarded for reporting on the ground truth. An inference’s assigned reward should be a means of rewarding the truth, not rewarding a worker’s stake in the network.
However, reputers still stake because the network must have economic security. This stake is then used for determining the ground truth, which does not require any particular insight; it simply solves the oracle problem of incentivizing reputers to relay information honestly.
This ethos shapes how contributions are valued and rewarded in the Allora Network. Because network participants adopt different roles in Allora, they are rewarded through different incentive structures:
- Workers—They provide AI-powered inferences to the network. There exist two kinds of inference that workers produce within a topic: the first refers to the target variable that the network topic is generating; the second refers to the forecasted losses of the inferences produced by other workers. These forecasted losses represent the fundamental ingredient that makes the network context-aware, as they provide insight into the accuracy of a worker under the current conditions. For each worker, the network uses these forecasted losses to generate a forecast-implied meta-inference that combines the original inferences of all workers. A worker can choose to provide either or both types of inference, and receives rewards proportional to its unique contribution to the network accuracy, both in terms of its own inference and its forecast-implied inference.
- Reputers—They evaluate the quality of inferences and forecast-implied inferences provided by workers. This is done by comparing the inferences with the ground truth when it becomes available. A reputer receives rewards proportional to its stake and the consensus between its evaluations and those of other reputers.
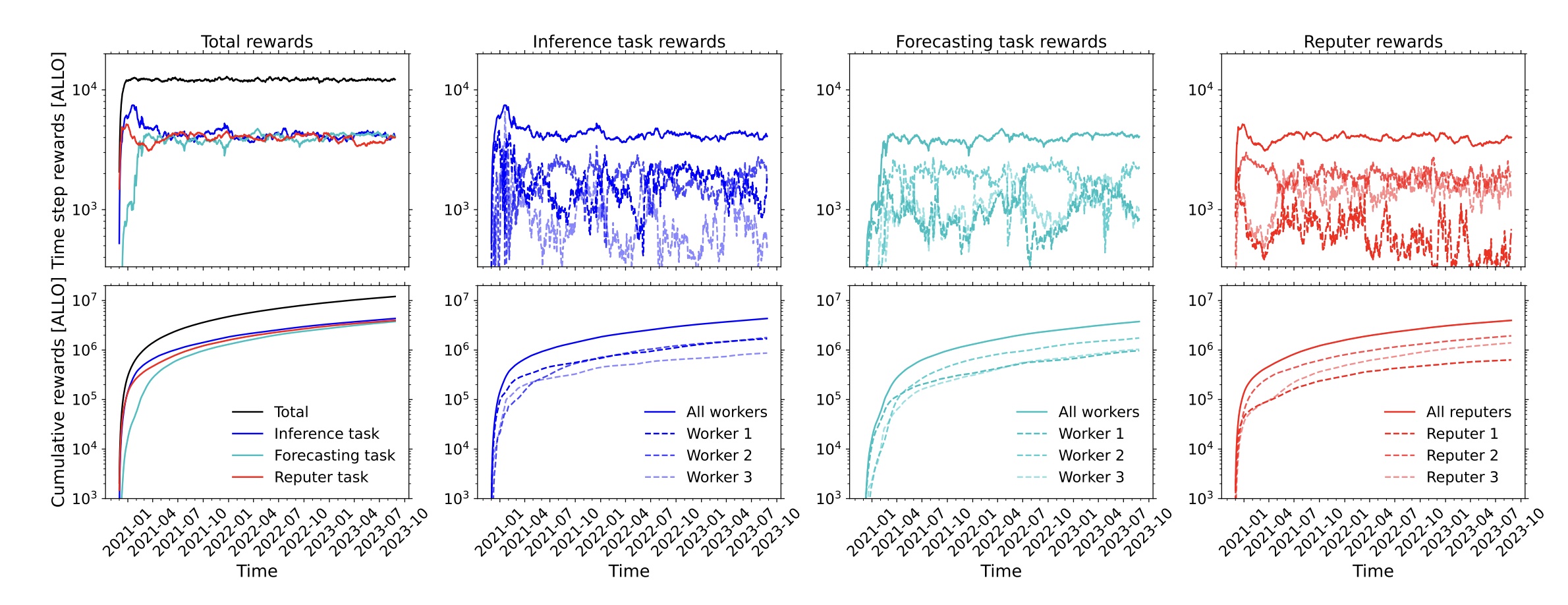
Because of this differentiated incentive structure between workers and reputers, the network optimally combines the inferences produced by the network participants without diluting their weights by something unrelated to accuracy. This is achieved by recognizing—and rewarding—both the historical and context-dependent accuracy of each inference.
Allora’s collective intelligence will always outperform any individual contributing to the network.
The original mission in creating Allora was to commoditize the world’s intelligence. The innovations of context-aware inferences and the differentiated incentive structure address two major challenges that make that mission possible.